Taking AIM at Data fusion (part 1) Why Advanced Information Modelling (AIM) matters to the businesses of today (and tomorrow)
- Elemendar
- Sep 10, 2024
- 4 min read
If you haven’t heard of it, the challenge of ‘data fusion’ is an increasing issue for how businesses manage and process their data and information. It is crucial as part of how businesses maintain and support their own high-value business intelligence to support their ongoing commercial success.
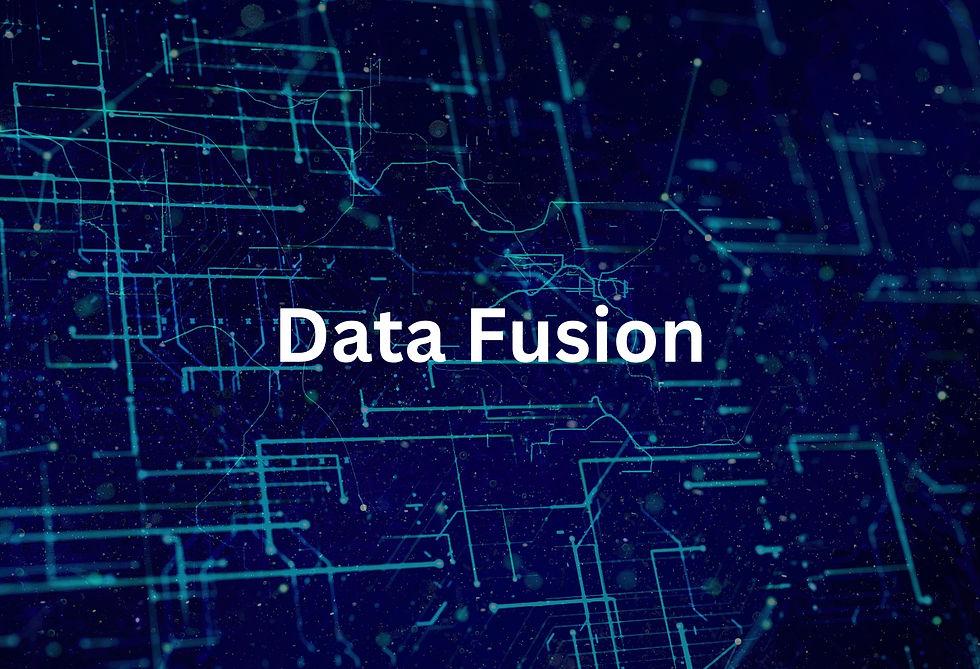
Put simply, data fusion describes the process of combining data from multiple sources to create a single source of information that is superior to its constituent parts. It is a key component of business reporting or dashboarding in order to support decision making or further business analytics. At Elemendar we specialise in leveraging AI and advanced information modelling (AIM) to help address this challenge. This blog here provides an example of how we have applied AIM in practice to a sample CTI investigation, but before then, it is useful to give a more basic use case on why Data Fusion matters to your business.
Data Fusion in business (sample use case)
Dyno-Freight is a made-up company that works out of the UK. Although it is a UK company it has operations based in the UK, France and Germany each with separate fleet management systems to handle company vehicles.
Dyno-Freight has grown successfully over many years and along the way acquired similar, smaller companies in France and Germany. As a result they support their own internal information management systems as well as French and German systems that were inherited from historically acquired companies and whilst modernised over the years, each system is still somewhat bespoke to the operation from which they originated. As a result, Dyno-Freight manages systems that are similar but do not hold exactly the same types of data.

Figure 1: Comparison between the Imperial system and the Metric system, highlighting units such as miles, pounds, kilometers, and euros.
Why does this matter?
The UK Head Office is reviewing their vehicle management policies and would like information relating to the company-wide use of vehicles to support their decision making and address new legal requirements for emissions. This includes metrics such as average monthly mileages covered, average (manufacturer-stated) fuel economy and various costs.
This creates an immediate challenge for the UK Head Office team - they must gather and integrate data from all 3 systems and report as a whole to the company’s board. In doing so they confront many complications - including the fact that the UK system uses imperial units and Pounds Sterling for costs whereas the French and German systems use metric units and costs are in Euros.
Additionally, the German system does not hold fuel economy figures for their vehicle types as it was never relevant to their operation. However, the French database contains this data for all vehicle types used in both France and Germany although it is expressed in litres used per 100 kilometres versus the UK's miles per gallon!
For our made-up company, this provides an example of a typical data fusion scenario where we must integrate several sources of data, use one source to fill gaps in another and normalise units in order to present a single set of harmonised metrics.
How does AIM address this?
Fortunately for Dyno-Freight, their UK, French and German fleet management systems have been structured according to AIM principles. Specifically, by following the ‘ontological approach’ encouraged by AIM means that the data has already been structured in terms of the concepts required for the Head office team to make their reports. They have developed and used a specific ontology that provides a common framework to describe their data e.g. vehicles, insurance organisations, costs or emissions.
The development and use of a defined ontology guides developers down standardised paths for data representation. This means that before the requirement for the head office report was generated, an ontology had been developed to put all different sources of data into the same format. The result being that the systems (i.e. those developed and supported in the UK, France and Germany), despite being maintained independently, are able to represent the data in a similar manner which makes integration much less complicated.
If we consider the sticky issue of unit conversion as well (i,e, kilograms to pounds, meters to feet) the ontology also mandates that quantities are coupled to their units of measure. So, Dyno-Freight’s distance figures whether in the UK, French or German system, will always be accompanied by the unit used e.g. miles or kilometres. This clarity prevents errors caused by the ambiguity of bare numbers e.g. ‘distance: 50’. There are many historic examples of engineering projects failing due to failed assumptions or conversion errors around different units of measurements being supplied - the NASA Mars climate probe failure in 1999 is an often quoted example of this - here
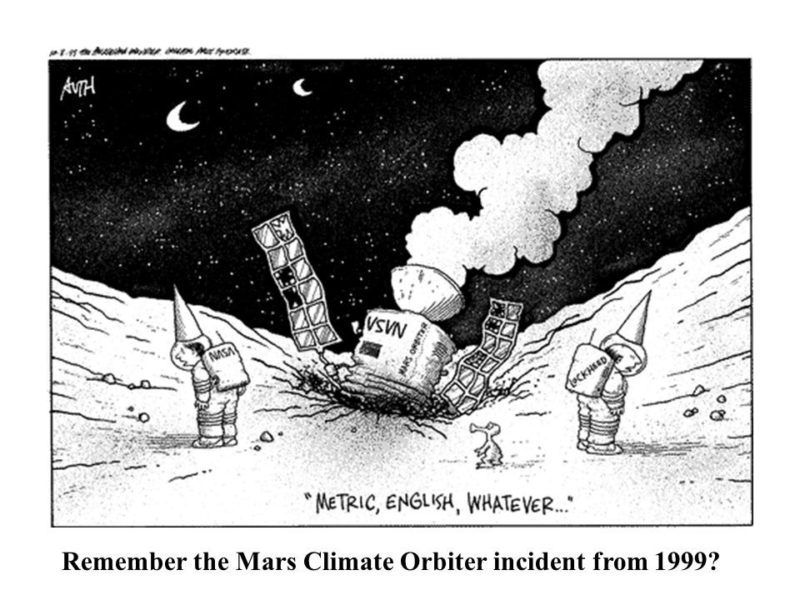
Figure 2: Newspaper cartoon depicting the incongruence in the units used by NASA and Lockheed Martin scientists that led to the Mars Climate Orbiter disaster. (Source: Slideplayer.com)
At Elemendar, we are also further advocating the benefits that Ontological representation brings as it can further support the challenges caused by data fusion by making it possible to use machine learning for automatic entity extraction/integration. So if you consider our conversion issue, AIM provides the opportunity for better standardisation of different sources of data and information using automated tools. In theory, following this and using a foundational ontology there are also the structures and frameworks in place to enable automated conversion of data at source - so bulk conversion of different units into the required format could be handled as part of the data fusion process. To enable these benefits, Elemendar is helping form a 10 year roadmap for using AIM to address data fusion.
About us
What is AIM? Over the past 30 years, the UK research community has pioneered a new database technology built upon our understanding and collective research on Advanced Information Models (AIM). As a key partner in this research, Elemendar has played a key role in bringing together and working with this rich community of operators who have built specific AIM techniques. In addition, through its engagement through the NCSC accelerator, Elemendar has pioneered the application and development of specific 3d and 4d Ontologies for Cyber Threat Intelligence, please get in contact if you would like to know more about this research.
Acknowledgements
This blog post was authored by:
Chris Evett, Head Of AIM
Ross Marwood, AIM - Technical Architect
Data fusion is important and hard, definitely a worthy aim
Very interesting, thank you, looking forward to read part 2🤓