Taking AIM at Data Fusion - Part 2 - Data fusion for Cyber Threat Intelligence.
- Elemendar
- Sep 19, 2024
- 4 min read
In part 1 of this blog, we introduced the concept of data fusion and how Advanced Information Modelling (AIM) can help. If you haven’t heard of it, the challenge of data fusion is an increasing issue for how organisations manage and process their data and information, in order to maintain and support their own, high-value business intelligence.
The following example using AIM for a structured cybersecurity threat investigation can help with understanding data fusion, both in practice and as part of Elemendar’s ongoing mission to make intelligence actionable. This use case was produced as part of research activity conducted by Elemendar and with its partners in DSTL, DASA and the wider security community.
Applying AIM to Cyber security risk analysis
For this example of data fusion, relevant Cyber Threat Intelligence (CTI) data was collected by a mixture of human and automated tools from the most relevant sources and processed into some form of recommendation, most likely to the security team and in the form of a risk assessment. Specifically, a key vulnerability to the national infrastructure supply chain was identified as follows:
“The 3CX supply chain attack keeps getting worse: Other vendors hit” - 3CX Software Supply Chain Compromise Initiated by a Prior Software Supply Chain Compromise; Suspected North Korean Actor Responsible
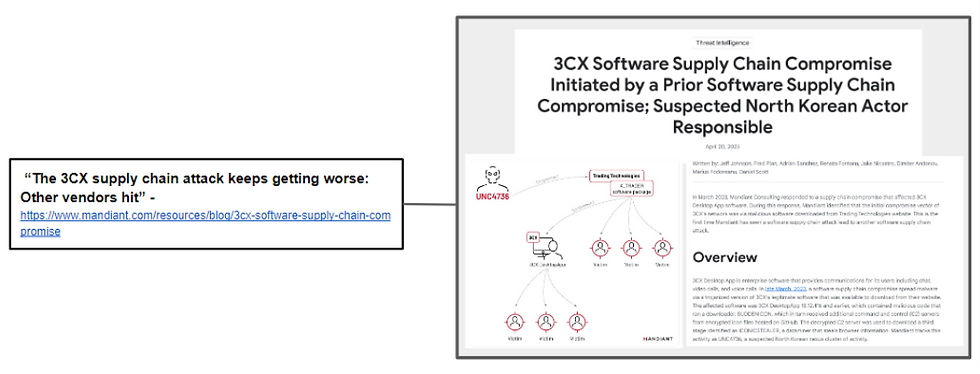
In this example, to understand this risk better a human analyst collected 30 relevant CTI reports from a range of different sources and in various formats.

How does the analyst then process this information to produce relevant insights and make recommendations?
In this investigation, the analyst then took these 30 pieces of intelligence and subjected them to different forms of analysis:
Manually assessed documents from the investigation
Elemendar’s bespoke PDF Tabular Entity extraction system, that automated the bulk extraction of relevant data from tabular fields in the intelligence documents
Elemendar’s READ application, to analyse and extract specific threat intelligence information as STIX outputs.
As a result, this investigation generated three different types of output from these three different forms of analysis.
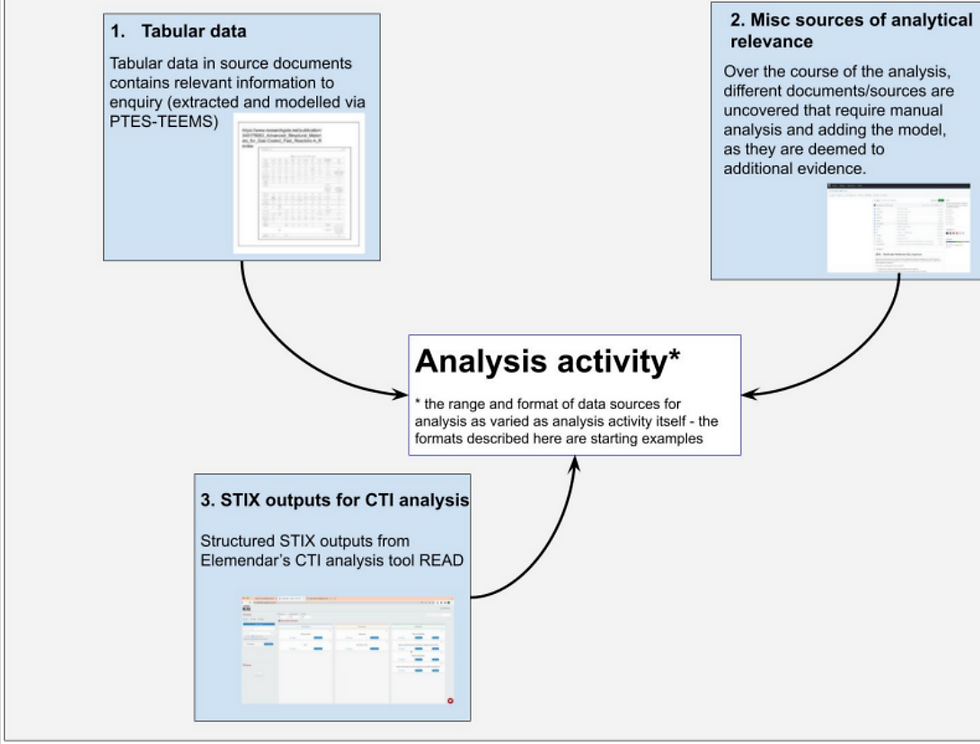
How can AIM help this example?
AIM addresses such diverse analytical activity by applying a standardised, ontological approach. For those unfamiliar with the term, an ontology is (put simply) a set of concepts and categories that allows data relating to a particular phenomenon (or subject of interest) to be expressed with specific properties and relationships.
Considering our CTI example, rich ontologies do exist as well as further underlying frameworks and analysis techniques. Industry standards such as STIX enable a taxonomy for how we organise and take action on CTI. For the purpose of this blog, we have simplified this big, complex area to consider only a few particular entity types relevant for specific actors common to this form of analysis. Having examined the original manual modelling of the 3CX supply chain attack, the following specialised ‘types’ were chosen to be created in the ontology. By using an ontological approach, we defined these key entity types and applied them across all three analytical sources. Some entity types of particular note to the investigation (across all of the sources) were as follows:
Basic Entity Type | Analysis example |
Organization | Lazarus Group |
Organization | CrowdStrike |
Association | Attack Attribution Association |
Role | Attacker Role |
Role | Attributor Role |
Role | Victim Role |
What benefit does this unifying or ‘Top Level’ Ontology bring?
For this example, the ontology applied allowed a combined model to be produced for all of the three analytical sources considered; this is called a Top Level Ontology (TLO). The TLO could then be directly queried by analysts to extract the relevant information from all three sources combined.

What are the benefits of this approach?
Although still relying on some manual aspects of conversion and ontology generation, this research illustrated how an ontological approach (specifically using what is known as a ‘4D’ ontology) allowed the relevant intelligence products to be combined in one queryable model. Future capabilities aim to automate and support the bulk formation and conversion of larger and more complex data stores and further address the challenge of data fusion for the business.
Please note, for more details on what a 4D ontology is and how it contrasts with conventional ‘3D’ ontologies used to build most databases today please have a look at ‘relevant technical details section’ the end of this post.
Final thoughts
The purpose of this and the previous blog post has been to illustrate the value of AIM to data fusion - an ongoing challenge to all organisations today. A technical deep-dive into the specifics of AIM and the use of ontologies will follow in part 3 of this series.
Please contact us if you have any thoughts or ideas on either the challenge of data fusion to your organisations, or how AIM can be used in practice.
Detailed Technical Information - what is a 4D ontology?
A 4D ontological model goes beyond modelling particular aspects of data including entities, types, relationships and individuals (often conventionally represented in a ’3D ontology'). It also models the temporal states of those things (hence the application of the term '4D'). For example an entity can have a particular relationship with another entity (e.g. an employee within an organisation) and each of the entities and the relationship itself can have a ‘state’ that persists for a specific period of time. The 4D ontology seeks to model all of those aspects including the state of the object (or associated relationship). For more details on this please see the following ref - M. West, Developing High Quality Data Models. Burlington, MA: Morgan Kaufmann, 2011.
About us
What is AIM? Over the past 30 years, the UK research community has pioneered a new database technology built upon our understanding and collective research on Advanced Information Models (AIM). As a partner in this research, Elemendar has played a key role in bringing together and working with this rich community of operators who have built specific AIM techniques, and pioneering the application and development of specific 3d and 4d Ontologies for Cyber Threat Intelligence. Acknowledgements
This blog post was authored by:
Chris Evett, Head Of AIM
Ross Marwood, AIM - Technical Architect
Great summary of the promise of AIM. Good to see it being used in practice for cybersecurity applications.
thanks very informative!